Do you know what the word “peruse” means? You may have used it in the context of “perusing a menu” signifying you’ve casually skimmed it. But in fact, the primary dictionary definition is “to examine or consider with attention and in detail,” which is the literal opposite of how many people use it in our day-to-day.
The phrase “actionable analytics” is a lot like that. As it becomes more and more common in the business world, especially in SaaS, the fundamental meaning of the phrase has been getting lost. Suddenly any type of data is being labeled as “actionable analytics,” even if that’s not the case. And while the definition of words like “peruse” can shift and morph over time, technical phrases that describe specific processes and theories shouldn’t.
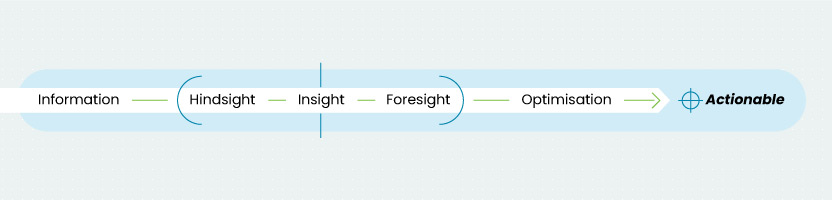
So how should actionable analytics be defined? What does a more specific definition of this phrase mean for your business? Let’s dive in.
The Elevator Pitch for Actionable Analytics
Actionable analytics is the process behind moving customer behavior analytics from purely informational to actionable, through phases that strive for proactive foresight, and not just predicting what will happen, but eventually determining what will happen before it even does.
Visualizing the process of actionable analytics can make it easier to understand:
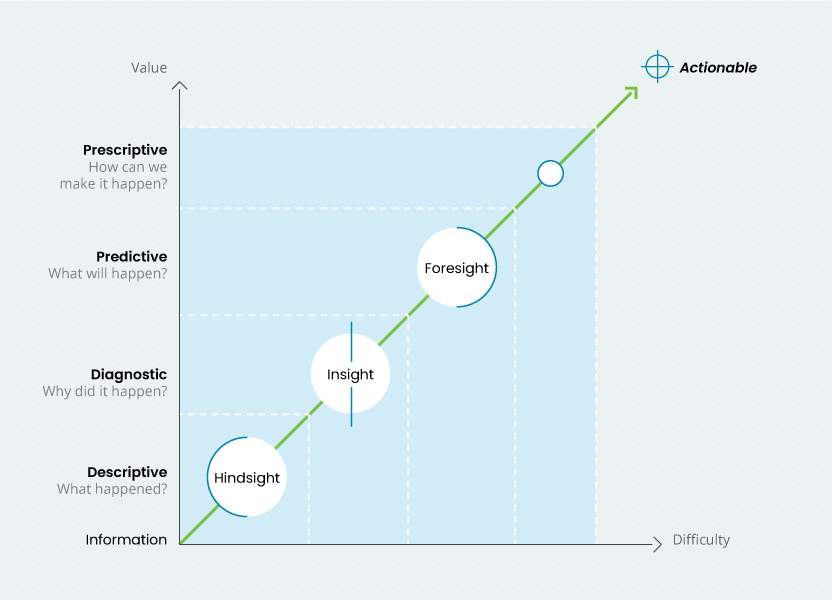
Actionable analytics encompass the progression through four phases: descriptive analytics, diagnostics analytics, predictive analytics, and prescriptive analytics. As a business progresses through these phases, their data can do more things, moving from providing hindsight to insight to ultimately foresight. As the data is optimized to be more useful, their analytics increasingly become more actionable.
Let’s take a look at each phase of this progression.
Descriptive Analytics
Descriptive analytics is purely informational, which is where the majority of today’s analytics providers live. This type of analytics shows a company what happened, providing hindsight to events that already took place and nothing more.
A great example is Google Analytics.
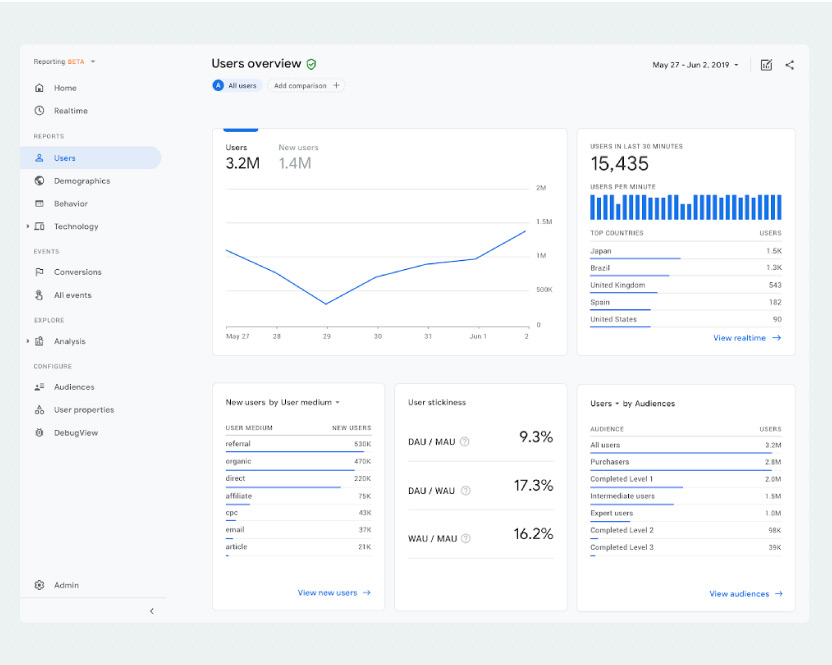
In essence, descriptive analytics is the bare minimum of simply gathering data, even if that data is complex and hard to collect. Machine learning algorithms that engage in data mining can be extremely powerful and thorough, but the output only tells you what is happening, not why.
At this stage, the data itself is only actionable when interpreted by an analyst who can parse the data into patterns and ultimately into recommendations. That’s not to say that some of the data can’t be obvious and immediately actionable of course. It doesn’t take much analysis to determine that zero traffic and a 404 error on the homepage are related, for example. But deeper insights into what your business should be doing next will not be clear at this phase.
Diagnostic Analytics
The next phase in data optimization is diagnostic analytics, providing the why something happened in addition to just the what. This next step is where most customer behavior analytics tools stall out, unable to provide businesses with the real reasons behind data trends and patterns. Traditionally, this phase is when an in-house data scientist will have to take the hindsight gathered and perform her own diagnostic.
Newer platforms on the market, such as InnerTrends, use machine learning algorithms to diagnose the growth opportunities that the raw data presents.
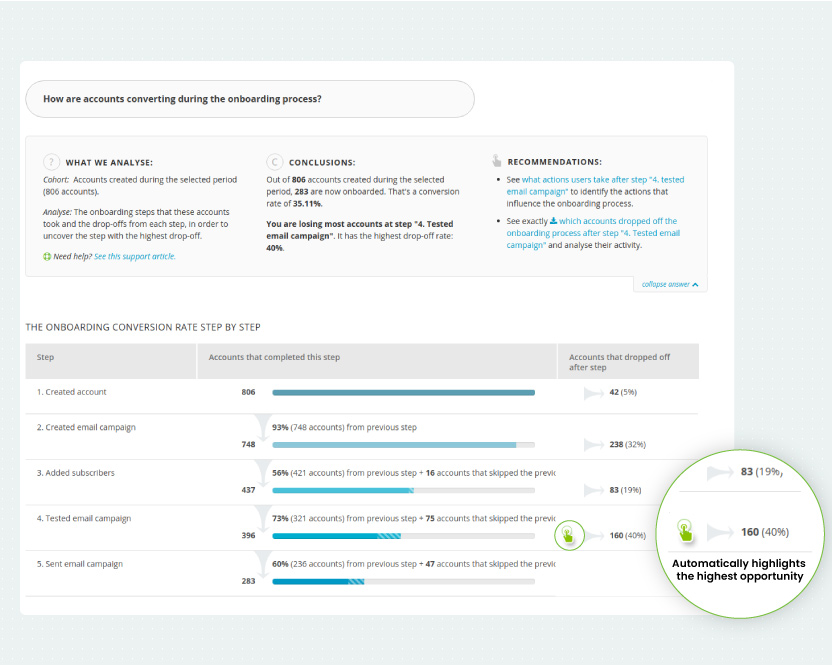
For example, a diagnostic analytics tool can interpret the data of a company’s onboarding conversion funnel and automatically determine that step 2, not step 1 or 3, of the funnel is where the greatest growth opportunity resides.
In other words, instead of you telling the analytics software what your Key Performance Indicators are so it can place them on a dashboard for monitoring, the software itself keys the right performance indicators for you in order to find opportunities you wouldn’t have found yourself.
This type of automated diagnostic analytics can be incredibly useful for businesses who may not have the resources to retain a data scientist, nor the time to pour over their thousands of data points themselves.
Predictive Analytics
Predictive analytics goes beyond the what and why to the what’s next? Using predictive models from the data to forecast what is likely to happen in the future, this phase can tell companies what may result if certain actions are taken today.
A recent and profound example of predictive analytics in action was in forecasting the spread of COVID-19 across the country and internationally. Researchers and data scientists worked to create predictive models of the future spread of the virus based on complicated data points such as infection rates, population density, vaccination rates, and more to provide better decision-making for healthcare providers in those areas.
Businesses can use predictive analytics to play with specific “levers” to see what the projections may be. What will the onboarding conversion rate be if more emails are sent in the first week? What if the onboarding videos were each half as long? What if the initial onboarding phase was extended another week out? Data modeling can tell you the predicted outcome of pulling each of these levers so you can take the most impactful actions.
Prescriptive Analytics
This fourth phase of actionable analytics is the aggregate and culmination of the prior steps. By gathering good data to document what happened, understanding why it happened, and predicting what may happen next; prescriptive analytics provides insight into what companies can do today to make desired results happen tomorrow.
A good example of this in practice that we all probably have experienced is how Amazon makes you buy certain products. Note, that’s make not suggest.
Using its millions of data points gathered on not just you, but the hundreds of thousands of shoppers like you, Amazon can tell that you are, say, moving into a new apartment and will probably be shopping for a new bookshelf. Knowing this, and knowing the behaviors of similar shoppers, Amazon’s algorithms can show you bookshelves that you have a high probability of purchasing (based on color, price range, etc) and chase you around the internet with ads. Amazon isn’t passively suggesting an array of bookshelves for you to choose from; rather, it’s using prescriptive analytics to make you buy from the ones it deems best for your needs and wants. Repeat this millions of times over, and you get the shopping juggernaut that seems to be reading all of our minds.
Prescriptive analytics is data at its most actionable, modeling your data to tell you where to spend more of your company’s resources to maximize your desired results.
So now that you have a good sense of what actionable analytics looks like, what can you do to make your data more actionable?
The Path to More Actionable Data
In general, there are three paths to making your data more actionable.
The first is to layer AI and machine learning on top of your data. Using algorithms that invoke techniques like clustering (ie. finding and grouping similarities in the data), the areas of your business that are in most need of attention can become more obvious. This is the strategy that many analytics tools on the market take.
Unfortunately, this method is only doubling down on descriptive analytics. Basically, by taking the human element out of it, these analytics tools use AI to diagnose issues smarter and faster. Patterns in the data are recognized in minutes instead of weeks. While this can greatly improve data quality and speed up aggregation, thereby making data more obvious, it only tangentially pushes toward diagnostic analytics. Someone in-house still has to look at the output and make suggestions on what to do next.
The second path is to get to a deeper understanding of your business in terms of data. Instead of collecting all the data you can to look for patterns that could help your business, you would first put effort into defining the metrics that matter most for the growth of your business, and then tailoring the data reports around those metrics.
An example of this is taking the time to define a company’s North Star Metric, which is the tipping point in the product’s usage that guarantees growth. Once defined and tested, the data can be used to optimize this metric. And since the goal of the data is simple and clear, it’s easy to see how the data has now been optimized to be more diagnostic than just descriptive.
Both these paths are fine on their own, but the third path is what will truly push data into being more predictive and eventually prescriptive, and thereby more actionable: a combination of both.
Taking the time to understand the most impactful metrics of your business, then layering on AI to analyze data patterns faster and with more efficiency, is the more difficult path but will ultimately add more value to your analysis.
So now that you know what path to take toward more actionable analytics, what are the necessary elements that every business needs to make this happen?
The 3 Components Needed to Make Data More Actionable
Insights from your data do not come from simple reports. Insights come from correlating the data from multiple reports and building a story out of those reports. This means that every insight needs to work with multiple data sets and depends on your ability to create a story out of all those reports that are aggregated.
An actionable insight is basically a story you can easily relay to your team that ends with what to do next. And there are generally two ways to get to the story: one, utilize an experienced data scientist to analyze the data and translate it into actionable insights; or two, utilize code to apply advanced algorithms to find patterns and build that story.
Either way, the components needed to pull actionable insights from your data are the same:
- Data Tracking
- Data Querying
- Data Algorithms
Let’s examine each of these components in closer detail, understanding how the majority of companies perform each one, and how they could be better optimized.
Tracking The Right Data
Collecting data should obviously be precise and accurate, but it’s one thing to simply dump all your data in a database, and another to track your data in a way that is useful. In some studies, more than half of the data a company collects is never used. Why does this happen?
Typically, this is how data tracking goes: a company installs a product or service that tracks data. That company comes up with a tracking plan that clarifies what metrics to track and the methodology used in the tracking process. This tracking plan lives on a document that serves as a reference for the company, including the development team that will implement the proper tracking code. Once set, analysts can log into the installed analytics service to interpret the collected data.
Unfortunately, this approach to data tracking runs into various problems. For one, different people at the company will have different tracking plans as they are interested in different metrics; these tracking plans will be biased and won’t easily align with each other.
For another, these tracking plans are often not based on clearly stated business needs and the stages of the customer lifecycle journey. Since some customers will be recently onboarded, some have been around for a year, and some aren’t even customers yet and simply trying a demo of the product; the data tracking plans rarely account for these very different user profiles which all have unique goals and success metrics.
Remember, for data to be actionable, the user behavior has to be clearly defined from the start so that it will be easy for your team to understand the patterns that appear as the user behavior data is collected.
Thus, better data tracking starts with defining your business from a data-driven perspective with the agreement of your entire team. We call this framework Business Concept Definitions, a process that aligns the company from the very beginning on the definitions of what your customer acquisition is, what onboarding is, and on through activation, retention, revenue, and referral stages. As each of these metrics are clearly defined, the data tracking plan can be geared toward tracking with more precision, making the eventual analysis that much easier.
Querying The Data
The second component of making data more actionable is your team’s ability to query the data, or rather, query the desired data from all of the metrics collected. Many analytics tools on the market today tout all the ways to slice and dice the data with dozens, if not hundreds, of ways to filter, segment, and cohort.
But unless the members of your team are experienced with each of these dimensions (which can verge on needing to be data scientists), the wrong queries can lead to wrong conclusions. Here’s a classic example:
Say you want to know how many of your customers finished the onboarding process that they started last month. Typically you would pull a report showing the accounts that signed up in a specific period of time, here the previous month. But the right report to pull may not be obvious. Pulling a segmented or filtered report, you would miss the customers that finished onboarding on the 1st day of the next month, even though they should be counted since they started the process last month. The issue is that you should have pulled a cohort report, not a filtered one.
This sort of querying issue happens frequently, not because the team is poorly trained, but because it’s simply not intuitive. We shouldn’t have to acquiesce to the complicated language of the tool we are using to query our data; the tool should acquiesce to our language.
One way to have more intuitive data querying is to translate advanced queries into plain English questions.
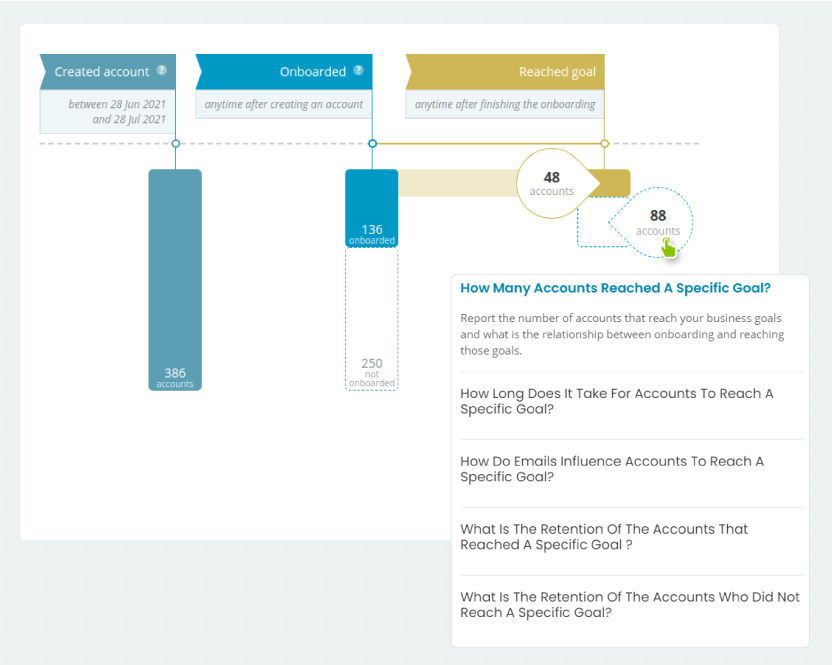
Intuitive data queries do not necessitate your team knowing how to manipulate any of the metrics, dimensions, segments, or cohorts. By translating queries into questions, the specific insights your team needs in order to take action can be pulled up by anyone, regardless of their data science acumen.
Remember, we started with better data tracking that narrowed down the metrics that are most important. Now with intuitive data querying, we can quickly find the actionable solutions that are in that limited data set. In other words, once the data is good and trustworthy, querying the data in plain English makes it easier to find the right insights.
Applying Algorithms To Find Insights
A single report is rarely able to tell the whole story. Most often, the story of your data is made up of multiple reports that are used to provide the context for the problem your team is trying to solve with analytics. This is where data algorithms come in, giving your team the ability to intelligently aggregate data queries into a cohesive story leading to actionable insights.
For example, a query of the data may show that the majority of the drop-offs during the onboarding process happens on a certain step. That’s good information to know, but why are they dropping off? Your good data tracking has amassed this information and your intuitive queries have honed in on it, but it’s by applying algorithms on the data that will provide the actionable next step.
They can help you solve the smaller questions: how long does a user spend at that onboarding step? What actions do successful users take between that step and the next one? What are the user actions that are likely to influence someone from finishing the onboarding process? Which then leads to answering the big question: what should I do next?
This analysis is difficult to perform unless your team has training in data science, which is why analytics tools like InnerTrends layers on these types of advanced data algorithms on top of your data to do the analysis for you.
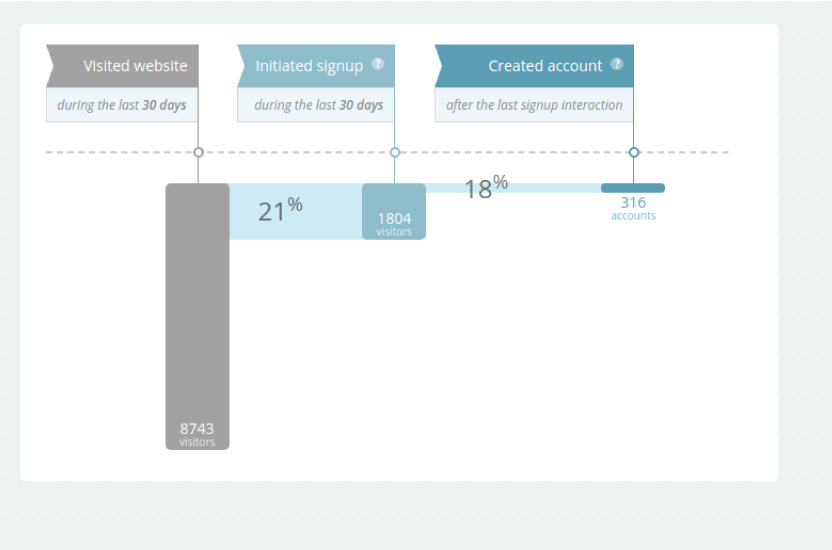
And as mentioned above, these algorithms help increase the speed of analysis as well, finding patterns and solutions that are not immediately obvious. It wouldn’t do any good to spend a month combing through the data to find out that it’s too late to apply the actions. Today’s data science can provide near real-time analysis to make the most impactful improvements to your business today.
So that’s what an actionable insight looks like. You start with high-quality data that is tracked with precision. This gains you the ability to create advanced queries on it, and to then apply advanced algorithms on that data, giving you the full story you need in order to take motivated actions for tomorrow.
Actionable analytics is a simple phrase to describe a complex and progressive journey that makes your customer data more useful for the success of your business. InnerTrends was built with this progression in mind, helping companies push toward a more prescriptive approach to putting data to use.
To learn more about what InnerTrends can do for you, click here for an interactive demo to explore our product.